Table of contents
Author: Ibrahim Dar
Article Speech recognition technology has been around since the 1960s. But it has never been as prevalent and useful to the average individual as it is today. From dictation programs to voice-recognizing language translators, speech-to-text is everywhere. So it makes sense for one to wonder how it even works.
In this article, you will discover the different uses of speech-to-text technology alongside the three-part loop in which it works. You will also discover its limitations and likely improvements. By the end of this post, you'll know 5 ways to use speech to text in your life. So let's get started with how it works.
Speech to Text - How does it work (3-part structure)
Speech-to-text technology works by cross-referencing voice data with the program's text library. Every word produces sound waves that are relatively unique to it. The sound waves, when converted into digital signals, also retain a somewhat unique signature.
The digital signal generated by converting "Hello" is different from the one generated by converting "Good Bye." As long as a program has learned what the digital signal of "hello" looks like, respond to hello by typing out the word. This isn't foolproof, though.
If you say you had a "hell of a day," the digital equivalent of the beginning of that sentence might sound like "hello" to the program. That's why context recognition and accent recognition are important. To understand this better, you must consider how humans understand speech.
When you say, “I’m sorry,” the sound waves heard by your spouse are different from those caused when you say, “You are overreacting.” Your partner’s reaction to those two utterances is also different. Humans react differently to words because humans have a library of words with which they match what they hear.
Humans don't need to convert "hello" into a digital signal, but they need to turn it into a neural signal that the brain can process. If they know what "hello" means, they can respond accordingly. And if they don't, they will ask for clarification.
On the surface, humans and computers seem to have a similar three-part speech recognition system.
Aspect | Human | Computer/Smartphone |
---|---|---|
Input | Received via ears | Received via microphone |
Converted | Into neural signals | Into digital code |
Processed | By cross-referencing with existing knowledge | By cross-referencing with a word-signal library |
The two key differences are that humans are better at context and accent recognition. When someone says "hails" because they aren't native English speakers, most people can tell that what they mean is "hello". Most speech recognition programs might not arrive at the same conclusion.
Similarly, when someone says that they're "dying to try something," most people can tell that the exaggerated emphasis is a show of passion. But computers might find it much easier to relate that word to "the Ying" because of the similarity of digital signals. Alternatively, a speech-to-text app might type "Dying and yang" when you say "The yin and yang".
So, most speech-to-text programs haven't been functional until the emergence of deep learning. With deep learning, speech recognition algorithms have started to learn context and even pick up on accents. That's why some speech-to-text programs are starting to replace human typists.
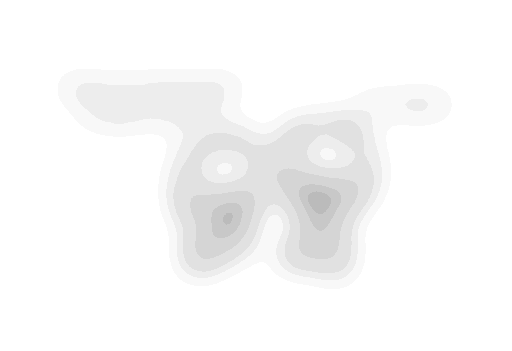
Speech To Text - Use Cases
Speech text apps that leverage deep learning and AI to go beyond word-matching have real-life applications that can disrupt a billion-dollar industry. Let's explore a few of the current uses of speech-to-text software.
Content Monitoring
The most common use of voice recognition is content monitoring. Platforms that are too big to handle for human moderators have machines do the job. And that’s possible only because machines can audiovisually treat content as text, thanks to speech-to-text technology.
Instead of human content moderators physically listening to the 500 hours of video uploaded to youtube per minute, the content moderating algorithm simply goes through the transcript of the videos and flags content for hate speech and violence. A human moderator can interfere at a later stage.
Speech recognition also helps Youtube figure out how to categorize content. A video that doesn't feature any mention of Johnny Depp will not rank for the search term "Johnny Depp News" just because the words are in the title. It is Youtube's way of getting around clickbait and misleading content.
Dictation
Moving away from content platforms and toward content creators, dictation is the most common use of speech-to-text. It is also the most straightforward use. Instead of taking notes by typing or writing them down, people can now take notes verbally.
Dictation also allows people to take notes on a walk, in a car, and during a workout. Because it takes less time and is stationary, and can be done more easily, many people prefer digital dictation over taking notes physically.
Voice Query
Dictionary naturally builds up to the next logical step: command. Now that search platforms and AI voice assistants work hand in hand, you don't need to type out your queries. Almost every home assistant works on voice commands alone.
Amazon Echo, powered by Alexa; Google Nest, powered by Google AI; and Apple HomePod, powered by Siri, are all home assistants that recognize your voice and process it as text. When you say, "Alexa, who is the tallest person alive?" your words are turned into a text query via Automatic Speech Recognition (ASR). Once the command is turned into text, the pre-established search technology handles the rest.
ASR has been a serious speed-up for voice technology. Because of ASR, Alexa, Siri, Cortana, and Google Voice, figure out your queries much quicker. There might be a time when there will be no "loading" time between your voice query and the results you get.
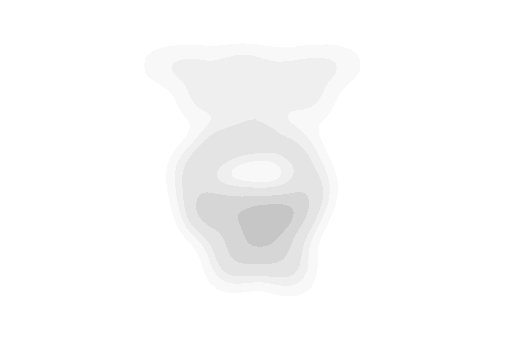
Transcription
For now, Automatic Speech Recognition and general speech-to-text technology are disrupting the transcription services market. Because machines are getting better at converting voice to text, human transcribers are becoming editors who flag mistakes.
And based on their feedback, AI voice recognition algorithms get better at nuance, context recognition, and even accent identification. In a way, the current generation of transcribers is helping algorithms get good enough to replace them completely.
Since most transcribers are assistants who transcribe minutes or take notes as a part of their job, AI is set to help them be more productive. As speech-to-text technology takes note-taking off their to-do list, they can play a more mindful role in their boss's enterprise.
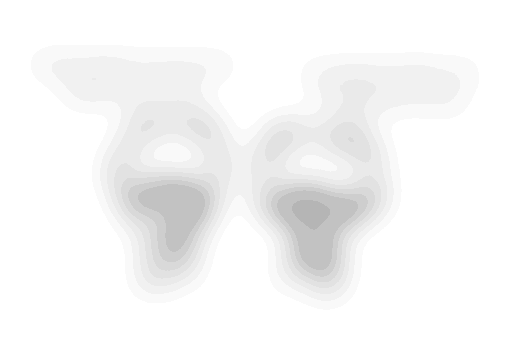
Speech To Text - Limitations And Potential Improvements
While speech-to-text conversion is one of the areas where tech has effectively overhauled human labor, it is still not perfect. There are several limits that prevent this technology from fulfilling its potential. And foremost among them is room for error.
Voice-Recognition Errors
As is the case with any AI-driven technology, mistakes are to be expected. Voice recognition technology has come a long way, but it is far from 100% accurate. That's why humans are required to prove AI-generated transcripts.
Not all algorithms are equally competent at voice recognition either. For instance, ContentFries's transcription accuracy is higher than the auto-generated captions of all major social media platforms.
Ultimately, voice recognition errors are quickly disappearing as limitations for speech-to-text technology. And it might soon reach a point where it makes as few transcription errors as human typists.
Accents
One of the major hurdles in AI speech-to-text technology becoming as good as human notetakers is accent recognition. A bulk of voice recognition algorithms are trained on American accents, making it harder for people from Asia, Eastern Europe, and even Britain to access their benefits.
Recently, voice-to-text services have come to realize the market potential of non-American accents. Still, major free speech-to-text services remain useless for foreigners. For instance, if you have an accent, automatic captions on pretty much every video hosting platform will misinterpret your words.
ContentFries keeps improving its accent accommodation, but the overall technology still has a long way to go before it becomes equally useful to global audiences as it is to Westerners in general and Americans in particular.
Library Limitations
A very serious problem with many speech-to-text services is also one faced by most print dictionaries: the pace at which our language is evolving. From "yes" to "dank" and "fam" to "finna," new words keep getting introduced to the social media sphere.
It isn’t a big deal if these words are absent from an academic transcription program’s library. But when an app that serves content creators cannot recognize “big yikes,” then it is indeed a big yikes!
Creator-driven technologies are better at lingo updates. ContentFries was built to serve the content repurposing model made famous by the most celebrated legacy content creator, Gary Vee. It is helmed by two deeply passionate individuals who want to serve the content creation market. So it makes sense that they can personally add new words they come across when consuming content.
But mass-use platforms that aren’t built around transcription don’t offer the same kind of up-to-date transcription.
Popular Speech To Text Apps And Programs
If there's one thing to pick up from the article so far, it is that the platform/app/service you pick defines how much you can benefit from it. Just like Google Bard and OpenAI's ChatGPT don't have the same performance, most speech-to-text programs aren't alike.
To avoid dealing with subpar, faulty, and inaccurate transcription apps, you must work strictly with market-leading services. Let's look at the popular speech-to-text apps that should be in your arsenal.
Speech To Text For Video Captions: Contentfries
This might sound like a self-serving suggestion because it is, but it is also a creator-serving one. ContentFries can be used to caption your content so it is more engaging and has a better chance of going viral. Because ContentFries' content repurposing technology revolves around accurate transcription, the company is incentivized to constantly improve its speech-to-text accuracy.
ContentFries isn't a traditional transcription app. It is a content-multiplying platform that has a transcription engine. You can transcribe and export entire blogs and articles from your webinars and podcast clips. But the most popular use case of ContentFries is short-form content creation.
Educators, podcasters, and entertainers use ContentFries to create well-captioned short-form content to post on TikTok, Reels, and Youtube Shorts. In an era of tiktokification, ContentFries is a very potent all-in-one content replication platform for many creators.
Speech To Text For Taking Notes: Otter.Ai
Traditionally, speech-to-text has been used to take notes and meeting minutes. In that aspect, Otter.ai is a market leader. It is a very accurate and easy-to-use platform where you can record your notes via your phone's speaker. It has a free version limited by recording duration. The paid version allows you to transcribe pre-recorded audio alongside live recorded content. This opens up the door to repurposing and even content analysis.
Speech To Text For Analysis: Whisper AI
From creating podcast timestamps to commentary videos, there are plenty of reasons you may want to see text transcripts of hours of content. Almost every speech-to-text service caps the hours of content you can transcribe, though. And that leads us to the main problem: feasibility.
ContentFries and Otter.ai are not feasible if you want to analyze over 1000 hours of audio. And many freelance podcast producers have to timestamp hundreds of podcast episodes each month. For professionals who need to transcribe thousands of minutes, installing Whisper AI might be worth it.
To get Whisper AI, you have to install 5 different items and must have some knowledge of Python. You can even invite a programmer to do it for you. Once it is installed, you will be able to transcribe and export thousands of minutes without a per/minute charge.
Speech To Text For Digital Assistance: Siri
If you want to use a digital assistant that can quickly catch and convert spoken word to text, there's nothing better than Siri. However, Siri is exclusive to Apple devices, so non-Apple users have to rely on alternatives like Alexa.
Speech To Text For Shopping: Alexa
Amazon Alexa is the perfect voice assistant for online shoppers. It is integrated with the Amazon ecosystem, or should I say Echo-system. If you order things online regularly, then Alexa's speech-to-text recognition will help you more than that of Google.
Speech To Text For Search: Google Voice
Google Voice is ideal for searching the web because it is integrated with Google, the world's largest search engine. The best part about Google Voice is that it is available on all types of devices, including the Apple iPhone and Android smartphones.
Speech To Text - A Content Creator’s Secret Weapon
Speech-to-text is used by consumers primarily to look up things and buy what they need without having to type. Students use the tech to take notes and analyze the content of lectures they have attended. But among those deriving the most benefit from this technology are content creators. Let's explore six ways in which content creators can use text-to-speech technology.
Repurpose videos to blog posts - The audio of your lecture, monologue, or podcast can have a substance that is perfect for a blog post. You do not need to type out the points you have made in a video when you can turn your video essays into essays via the ContentFries transcription engine. You can also use ContentFries' transcription engine for a range of content repurposing tasks, including quote card creation and converting audio to visual posts.
Create commentary content more quickly - Commentary content entails consuming content to comment on. But if you use speech to text, you don’t have to sit through hours of video before commenting on it. You can analyze the transcript and build your argument. Digital transcription can also help you analyze a large amount of content using online tools. Plenty of apps like MonkeyLearn can pick up patterns from text. By turning an audio into a digital body of text, you can see how often certain words are spoken, how the presenter strings together his ideas, and much more.
Timestamp your content more easily - Podcasts and long videos are better served with timestamps. A producer or an assistant will charge by the hour for this service. Text-to-speech technology allows you to create accurate timestamps in a few minutes.
Script your video essays more easily - If you find it hard to face the blank page, you can simply start talking and record yourself using a transcription app like Otter.ai.
Caption your content quickly - With apps like ContentFries, you can easily create and superimpose captions without typing them out individually.
Optimize your videos’ SEO - Your video’s substance is quite important for its SEO. Getting a text transcript of your videos and using them for content description can make your videos more discoverable.
Final Thoughts
Speech recognition technology has been around since the 60s. It is becoming more relevant now because of its value in the creator economy. By cross-referencing audio signals with a text library, the software can now convert speech to text, allowing computers to transcribe, interpret, and categorize audio. Content creators can use speech to text to technology for timestamps, content repurposing, and research.